AI's Impact on Cryptocurrency Fraud Detection
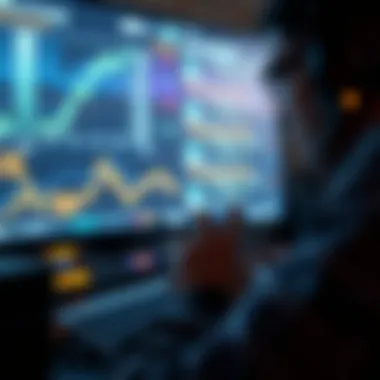
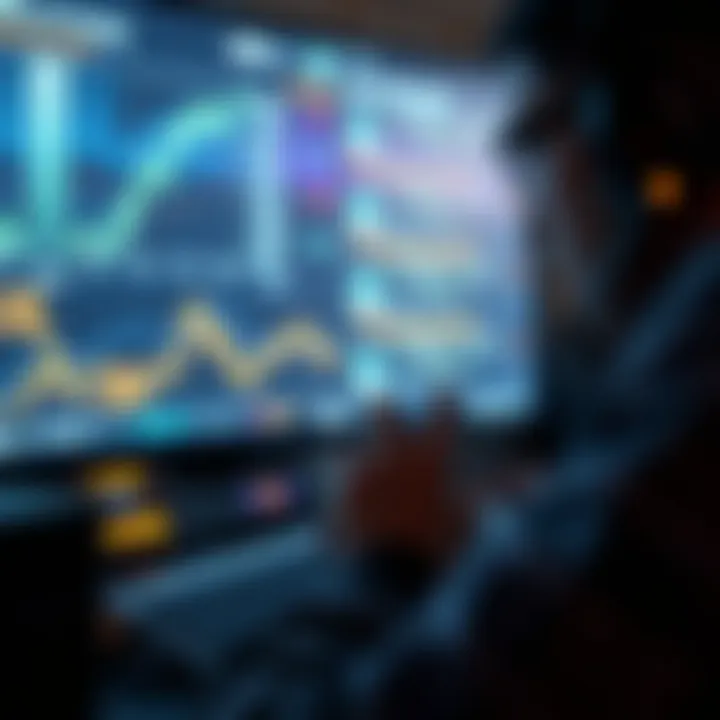
Intro
In the rapidly evolving world of cryptocurrencies, the need for robust security measures rises in tandem with the increasing popularity of digital currencies. Fraud detection has become a critical aspect, as both investors and the industry itself seek ways to protect against the ever-looming threat of scams and deceitful activities. This is where the integration of artificial intelligence comes into play, acting as a proactive guardian within this virtual financial realm. By harnessing the power of AI, we are capable of identifying sophisticated fraudulent schemes while simultaneously enhancing the overall integrity of transactions in the cryptocurrency landscape.
Key Concepts in Cryptocurrency
Understanding the foundation of cryptocurrency is vital when exploring the role of AI in fraud detection. Let's break down the essential concepts.
Definition and Importance
Cryptocurrency is a form of digital or virtual money created using cryptography for security. It operates independently of a central authority, making it decentralized. This inherent characteristic not only provides advantages like security and privacy but also poses significant challenges for tracking transactions and identifying fraudulent activities. Hence, effective fraud detection mechanisms are crucial for fostering trust and growth in this innovative financial space.
Blockchain Technology Overview
At the core of most cryptocurrencies is blockchain technology, which serves as a public ledger of all transactions. This distributed ledger makes it possible to maintain transparency and immutability of data, two key features essential for building trust. However, the challenge lies in figuring out which transactions are legitimate and which are not. Here, the intelligence provided by AI can be of immense worth.
AI algorithms can analyze the patterns of transactions on the blockchain, spot anomalies that may suggest fraudulent behavior, and initiate alerts before substantial losses occur. The combination of decentralized cryptocurrency with AI-enhanced fraud detection holds exciting promise for a more secure future in finance.
Trends and Innovations
Emerging Cryptocurrencies
As new cryptocurrencies pop up almost daily, each comes with its own unique set of features and technologies. Some focus on enhanced privacy, while others aim for scalability or interoperability. The diversity in these coins means potentially different fraud risks that need tailored approaches to detection.
Furthermore, the rise in decentralized finance (DeFi) has introduced novel vulnerabilities and risks, emphasizing the necessity for dynamic AI models that can adapt quickly to this shifting landscape. Leveraging AI can help improve user security as these new coins gain traction.
Technological Advancements in Crypto
The cryptocurrency world is also witnessing technological advancements that enhance fraud detection. From machine learning algorithms predicting potential fraud patterns to real-time monitoring systems powered by AI, the capabilities are growing. These technologies analyze vast amounts of data generated on the blockchain and can make sense of complex relationships that a human might miss. The ability to sift through transaction histories and detect questionable activities in milliseconds is a revolution in fraud prevention.
In summary, the intersection of AI and cryptocurrency is critically relevant as it creates a shield against fraud, allowing for a more secure transaction environment. As this technological synergy evolves, we can expect to see increased regulatory support and innovative applications that further enhance financial integrity. Understanding this landscape is crucial for marketers, investors, tech professionals, students, and analysts looking to navigate the intricacies of cryptocurrency today.
βAI-driven fraud detection stands as a testament to the melding of technology and finance, promising a safer future for digital currency transactions.β
For more insights, visit Wikipedia on Cryptocurrency, Britannica's Overview, and community discussions on platforms like Reddit.
Intro to AI and Fraud Detection
In the rapidly evolving world of cryptocurrencies, the intersection of artificial intelligence (AI) and fraud detection is becoming increasingly crucial. As digital currencies gain popularity, so do the methods that bad actors use to exploit vulnerabilities. Fraud takes many forms in the cryptocurrency landscape, and the stakes can be incredibly high. This necessitates the implementation of sophisticated technologies to safeguard transactions and maintain the integrity of the financial sector.
AI stands at the forefront of this technological safeguard, offering solutions that not only enhance transaction security but also streamline the detection of fraudulent activities. By applying machine learning and predictive analytics, AI can process vast amounts of data at an impressive speed. This capability is vital for recognizing patterns that may indicate fraudulent behavior.
Moreover, employing AI in fraud detection addresses the challenges posed by the anonymity of cryptocurrencies. Traditional methods of fraud detection often fall short in digital environments where identifying the origin of transactions is complex. The adaptability of AI technology to evolving threats makes it a formidable tool in the fight against fraud.
Understanding Artificial Intelligence
Artificial intelligence encompasses a broad range of technologies that allow machines to simulate aspects of human intelligence. This includes the ability to learn from experience, recognize patterns, and make decisions. In practical terms, AI leverages algorithms and statistical models to analyze data. Unlike traditional programming, where every scenario is explicitly coded, AI systems learn from data inputs, adapting their methodologies as they encounter new information.
In the context of fraud detection within cryptocurrencies, AI utilizes various techniques, including supervised learning and unsupervised learning, to categorize and interpret data. These approaches enable AI to detect anomalies that could signify fraudulent activities, refining its effectiveness as more data becomes available.
The power of AI lies in its capacity to sift through enormous datasets, identifying irregularities that may evade human detection. As such, AI enhances the overall robustness of fraud detection efforts, making it essential for users, investors, and professionals alike.
The Concept of Fraud in Cryptocurrency
Fraud in the cryptocurrency realm is a multifaceted issue, manifesting in numerous forms that often mirror what is experienced in traditional finance, but with a digital twist. The term "fraud" here encompasses various illicit schemes aimed at deceiving individuals or entities for financial gain.
Common fraudulent practices include:
- Ponzi Schemes: These promise high returns with little risk, enticing new investors to fund earlier ones. They rely on a continuous influx of new participants, making them unsustainable in the long run.
- Phishing Attacks: This method involves tricking individuals into providing sensitive information like private keys or passwords, often through deceptive emails or fake websites.
- Rug Pulls: A more recent phenomenon where developers abandon a project after raising funds, leaving investors with worthless tokens.
Each of these fraudulent schemes leverages the inherent vulnerabilities of digital currencies. The anonymity, lack of regulation, and sometimes opaque nature of transactions enable perpetrators to operate with a certain level of impunity.
"Understanding the nuances of fraud in cryptocurrency is paramount for implementing effective preventive measures and safeguarding investments."
The necessity to develop AI solutions tailored to recognizing and combating these fraud types cannot be overstated. With the rise of cryptocurrencies, both the risks and the need for innovation in detection mechanisms have never been more pressing.
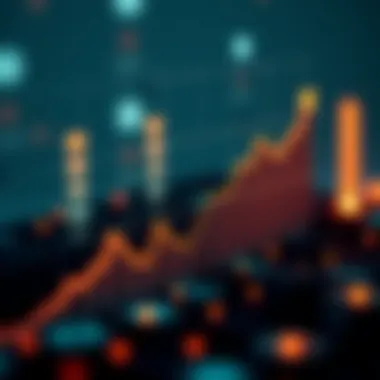
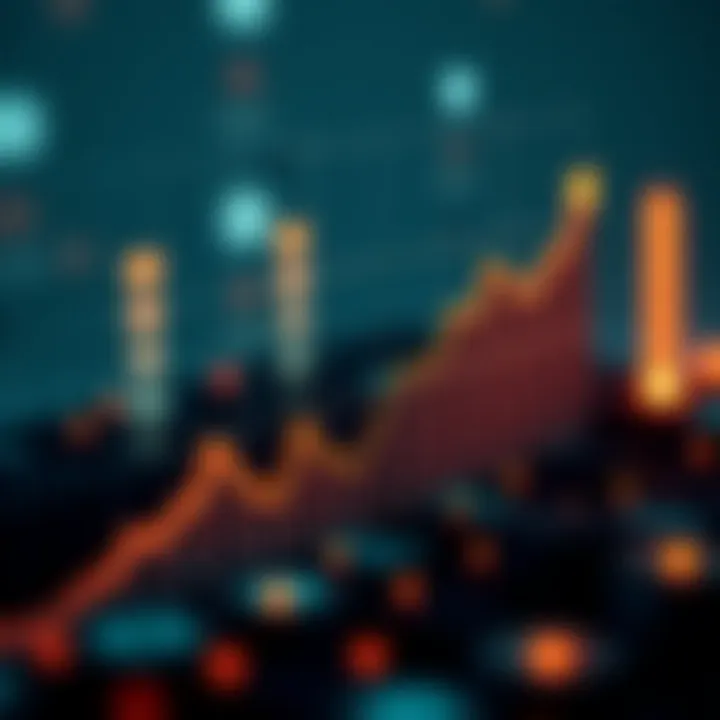
Significance of AI in Modern Financial Systems
In today's rapid-paced digital world, the significance of artificial intelligence in modern financial systems cannot be overstated. As the cryptocurrency landscape continues to evolve, the role of AI becomes increasingly pivotal. Not only does AI enable the automation of processes, but it also bolsters fraud detection capabilities, enhancing the overall security posture of the financial ecosystem.
Need for Advanced Security Measures
The cryptocurrency domain has witnessed a surge in the number of transactions and the influx of new players. This creates an environment ripe for fraudulent activities. Traditional security measures are often inadequate to combat the constantly evolving tactics employed by fraudsters. Here, the need for advanced security measures that leverage AI technologies becomes crucial.
- Sophistication of Threats: The methods used in cryptocurrency fraud are becoming more complex, utilizing social engineering and technological loopholes. AI-driven solutions can analyze vast amounts of data at lightning speed, identifying patterns that might elude human analysts.
- Real-Time Monitoring: Unlike older systems that rely on periodic checks, AI frameworks can provide real-time monitoring of transactions. By employing anomaly detection algorithms, unusual patterns can be flagged immediately, significantly reducing losses before they escalate.
- Data-Driven Insights: AI analyzes historical data, producing insights that help financial institutions proactively address potential threats. With the wealth of information at its disposal, machine learning can discern the subtle indicators of fraudulent behavior.
AI as a Tool for Threat Mitigation
AI's role in threat mitigation extends beyond simple detection; it is a comprehensive strategy intertwined with decision-making processes. Here are a few facets illustrating how AI acts as an effective tool in combating fraud in cryptocurrency.
- Predictive Modeling: By utilizing predictive analytics, AI can forecast potential fraudulent trends based on existing data. This proactive approach allows businesses to adjust their strategies and strengthen their defenses before specific threats manifest.
- User Behavior Analysis: Through natural language processing and machine learning, AI systems evaluate user behavior. For instance, if an account normally shows activity from a specific geographic region and suddenly executes transactions from a different location, the system can automatically prompt additional verification measures.
- Continuous Learning: A significant advantage of AI is its ability to learn and adapt. As new patterns of fraud emerge, AI systems refine their algorithms to ensure they remain effective. This cyclical learning process is key to staying ahead of fraudsters, who constantly alter their tactics to bypass traditional security measures.
"In the realm of financial integrity, AI is emerging as the beacon guiding institutions through the stormy seas of cryptocurrency fraud."
Blockchain Technology and Fraud Risks
Blockchain technology sits at the core of the cryptocurrency world, providing a decentralized and transparent system for transactions. The importance of understanding blockchain architecture cannot be overstated, as it influences how fraud occurs and how it can be detected. Each block in a blockchain records transaction data, forming a chain that is difficult to tamper with. This structural robustness offers significant promise for enhanced security against fraudulent activities. However, with every silver lining, clouds of risks still loom.
Fraud risks associated with blockchain span across various fraudulent practices. Despite the inherent transparency of blockchain, bad actors continue to find ways to exploit its advantages, leading to significant financial losses across the industry. To fully grasp these risks, we need to dissect common fraudulent practices that exploit weaknesses inherent to the blockchain landscape.
Understanding Blockchain Architecture
At its heart, blockchain architecture is fairly straightforward: it consists of a series of blocks linked together in a chronological manner. Each block contains a set of transactions that are verified by a network of nodes, ensuring consensus and integrity. The key characteristic of this architecture is decentralization, meaning no single entity has control over the entire network. This, in theory, should make blockchain resistant to fraud. However, this same structure can lead to challenges; a closed-loop, where bad actors could potentially collude to bypass the system's checks, is a significant risk. Moreover, the anonymity that blockchain provides can be a double-edged sword. While it offers privacy to users, it also enables criminals to hide behind a veil, making detection far more complex.
Common Fraudulent Practices in Cryptocurrency
Fraudulent practices in the cryptocurrency world often rely on exploiting the appeal of blockchain technology. Here are three prevalent schemes found in this ecosystem:
Ponzi Schemes
Ponzi schemes are perhaps the most notorious form of fraud in the crypto sphere. They promise high returns with little risk, luring in investors who might not be fully aware of the underlying mechanics. The key characteristic of Ponzi schemes is their reliance on the funds of new investors to pay returns to earlier investors. This can create a false sense of security and growth until the inevitable collapse occurs, leaving latecomers high and dry. A unique feature of Ponzi schemes is their ability to mimic legitimate investment platforms. They often utilize slick marketing and social media campaigns to attract hopeful investors. In this context, the ease of digital transactions and anonymity can make these schemes particularly enticing but also expose participants to significant risks.
Phishing Attacks
Phishing attacks pose another famous fraud risk, especially in the crypto trading realm. These attacks aim to deceive individuals into providing sensitive data, such as private keys or login credentials, through fake websites or emails. The primary characteristic that makes phishing attacks popular is their seemingly innocent appearance. They often imitate reputable exchanges or wallet providers. A unique feature of this fraud type lies in its adaptability; attackers continuously refine their methods based on current trends in technology and user behavior. While they may seem harmless at first, the consequences can be devastating, leading to complete loss of funds.
Rug Pulls
Rug pulls are a newer breed of fraud where developers abandon a project and take investors' money with them. The key characteristic of rug pulls is their suddenness and deceptionβprojects often appear legitimate until they execute the exit, disappearing with funds raised during initial coin offerings or liquidity pools. A unique feature of rug pulls is the charade orchestrated by the founders, which often includes extensive marketing to build up hype. This makes them particularly dangerous, given they initially entice investors with the promise of profitable returns. Like Ponzi schemes, they exploit the hope and naivety of investors, showcasing the critical need for robust AI-driven fraud detection measures to navigate this treacherous landscape.
Trust is critical in the world of cryptocurrency. Without it, fraud runs rampant, affecting not just individual investors but the overall integrity of the market.
Understanding these fraudulent practices is vital for anyone engaging in the cryptocurrency sphere. Awareness can drive the adoption of technologies like AI that enhance security and reduce risks associated with these deceptive operations.
Methods of AI in Fraud Detection
The integration of artificial intelligence in fraud detection marks a significant evolution in how financial transactionsβespecially in the cryptocurrency domainβare scrutinized. Traditional methods are often limited by human error and slow response times, while AI brings a level of precision and efficiency that is hard to match. By incorporating AI technologies, organizations can detect anomalies and fraudulent patterns that would otherwise fly beneath the radar. This section dives deep into the specific methods employed by AI in detecting fraud, focusing on machine learning algorithms, predictive analytics, and natural language processing.
Machine Learning Algorithms
Machine learning is at the forefront of AI methods used in fraud detection. It empowers systems to learn from historical data without being explicitly programmed. Each algorithm has its unique way of analyzing data which contributes to the overall goal of fraud detection.
Supervised Learning Techniques
Supervised learning is a standout in the toolkit of AI for fraud detection. By using labeled datasets, these techniques can train models to recognize signs of fraudulent behavior. Essentially, the algorithm learns from examples, much like a student learning from a teacher. The beauty of this method lies in its effectiveness: after sufficient training, a supervised model can spot fraud with remarkable accuracy.
One key characteristic of supervised learning is its reliance on quality labeled data; without a robust dataset, the performance will decline. This means cleaning and preparing data is crucial. A standout advantage is that once trained, these models can adjust to new patterns, which is especially beneficial in the ever-evolving landscape of cryptocurrency fraud. However, gathering and labeling data can be resource-intensive, and any bias in the data can lead to flawed results.
Unsupervised Learning Approaches
Conversely, unsupervised learning approaches operate without predefined labels. This helps in identifying unexpected patterns that could indicate fraud. It allows the model to look for intrinsic structures within data, which might not be visible through other methods. This is particularly useful in environments as dynamic as cryptocurrency where new types of fraud frequently emerge.
A key characteristic of unsupervised learning is its flexibility; the model can adapt continuously without the need for constant retraining with labeled data. This makes it a popular choice among organizations wanting to keep pace with aggressive fraud tactics. However, the downside is the potential for higher false positives, where legitimate transactions could wrongly be flagged as suspicious. Fine-tuning such models to balance sensitivity and specificity is a ongoing challenge.
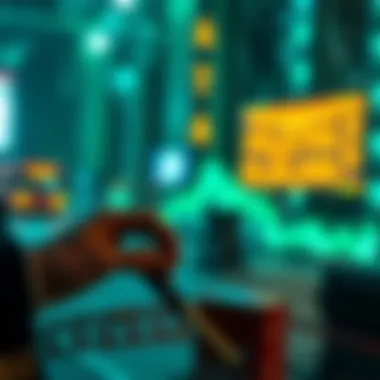
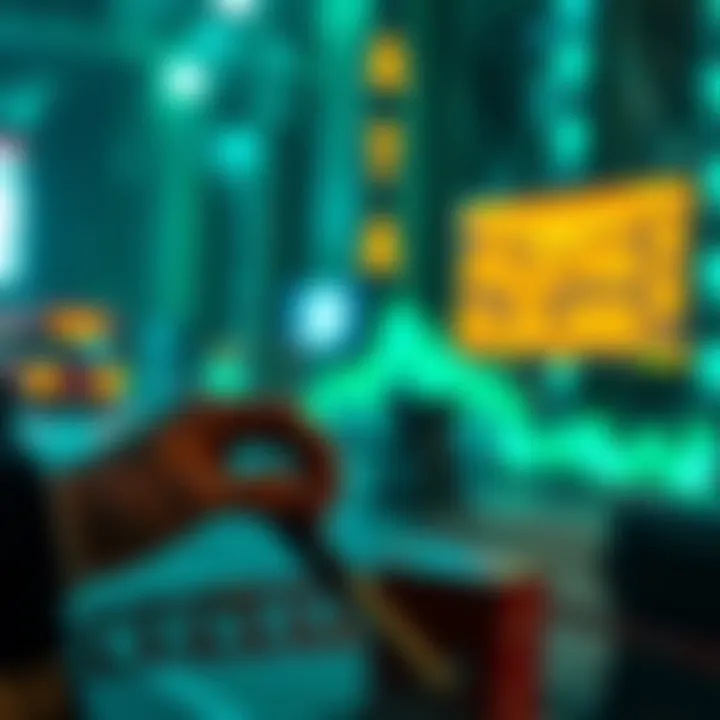
Predictive Analytics in Fraud Detection
Predictive analytics harnesses the power of historical data, statistics, and machine learning to forecast future outcomes. In fraud detection, itβs increasingly used to identify potentially fraudulent activities before they occur. Using a combination of various data points, algorithms can project likelihood scores indicating how likely a transaction is to be fraudulent.
Organizations often combine different data sourcesβtransaction histories, user profiles, and behavioral analyticsβto enhance their models. This multi-faceted perspective helps in formulating a comprehensive view of what constitutes normal vs. suspicious behavior. However, it's important to note that predictive analytics is not foolproof; external factors like market volatility and evolving fraud tactics can affect accuracy. Building a resilient predictive model demands continuous updates and validation to remain effective.
Natural Language Processing and User Behavior Analysis
Natural Language Processing (NLP) plays a pivotal role in analyzing user interactions across various platforms. The ability of NLP to understand text, sentiment, and context provides a nuanced layer to fraud detection. For example, in scenarios where communications precede fraudulent transactionsβsuch as a phishing attemptβNLP can process these communications and flag suspicious language patterns.
User behavior analysis complements NLP by studying how users engage with crypto platforms and services. By establishing baseline behaviors, unusual patternsβsuch as drastic changes in transaction size or frequencyβcan trigger alerts for further investigation. A significant advantage of employing both NLP and behavioral analysis is the comprehensive understanding they provide about usersβ interactions and intentions. Yet, implementing these technologies requires access to large datasets and robust processing capabilities to yield meaningful results.
Case Studies of AI Implementations
In the ever-evolving landscape of cryptocurrency and finance, examining case studies of AI integrations provides invaluable insights into real-world applications, successes, and challenges. These case studies serve as a reflection of the practical potentials and pitfalls of using artificial intelligence in fraud detection within this dynamic sector. By scrutinizing specific examples, investors, marketers, and tech professionals gain a deeper understanding of how AI can enhance security, mitigate risks, and drive innovation.
Successful AI Use Cases in the Industry
Several noteworthy examples highlight the successful implementation of AI in combating fraud within cryptocurrencies. For instance, Bitfury has been known for its advanced analytical tools that incorporate AI for transaction monitoring. By using machine learning techniques, Bitfury analyzes patterns and behaviors associated with various transactions on the blockchain. This not only aids in identifying suspicious activities more quickly but also enhances compliance with global regulations. Their ability to adapt AI models continuously based on transaction data underlines a dynamic approach to threat detection.
Another compelling instance comes from CipherTrace, a blockchain analytics company that utilizes AI to trace illicit cryptocurrency transactions. Their detection algorithms can analyze vast amounts of blockchain data to identify connections between wallets involved in fraud. This has proved essential for law enforcement agencies seeking to track down the origins of ill-gotten gains within the cryptocurrency space.
- Benefits of Using Successful AI Use Cases:
- Real-time Monitoring: Offers immediate insights into fraud potential, allowing for quicker responses.
- Adaptability: Algorithms learn from new data, improving their detection capabilities over time.
- Enhanced Compliance: Helps organizations adhere to regulatory requirements, mitigating legal risks.
Failures and Lessons Learned
While some AI implementations have flourished, not every attempt at integrating AI has been smooth sailing. A significant failure can be observed from the experience of a known cryptocurrency exchange, QuadrigaCX. The company encountered severe issues with its operations partly due to failures in its fraud detection strategies. Reports indicated that much of their system relied on heuristics that could not adapt to the evolving tactics employed by fraudsters. This resulted in substantial financial losses and ultimately contributed to the company's downfall.
Moreover, Coincheck, another exchange, was the victim of a major hack that exploited vulnerabilities due to insufficient AI-driven security protocols. The incident spotlighted the need for robust fraud detection systems that can withstand sophisticated attacks, emphasizing that merely having a system in place isn't enough.
Key lessons from these failures include:
- Investing more in sophisticated training for AI systems: Itβs essential to ensure that algorithms can evolve alongside tactics used by fraudsters.
- Regular audits and updates: Systems that incorporate AI must be continuously tested and updated to address new threats.
- Diverse data: Relying on a narrow data set can lead to blind spots that fraudsters can exploit.
"In the world of cryptocurrency, it's a constant game of cat and mouse. As one side innovates, the other adapts. Staying ahead depends not just on technology, but on understanding human behavior and motivations."
Through these case studies, stakeholders can appreciate the nuanced landscape of AI in fraud detection. They paint a picture of both triumph and tribulation, ultimately providing direction for future advancements in securing the cryptocurrency ecosystem. Keeping abreast of such case studies informs strategies not only for fraud detection but for overall security in the digital currency arena.
Regulatory Framework and Compliance
The regulatory framework surrounding cryptocurrency and its associated technologies plays a crucial role in shaping the landscape for AI-driven fraud detection. Establishing a robust set of regulations ensures that all participants in the cryptocurrency market, from individual investors to larger financial institutions, operate within a defined and secure environment. This minimizes risks associated with fraud and encourages innovation while maintaining accountability.
One of the most significant aspects of regulatory frameworks is the creation of guidelines that govern the use of AI in fraud detection. These guidelines help organizations understand what is permissible and what is not, thereby fostering a sense of security among users. When regulatory bodies set clear standards, it encourages entities to adopt AI solutions more willingly, knowing they are aligned with legal expectations. In this age of increasing cyber threats, such frameworks are vital to promote trust in cryptocurrency initiatives.
Global Regulatory Landscape
Navigating the global regulatory landscape can feel like trying to follow a twisty, winding road with many forks. Different countries have adopted varied approaches regarding the use of cryptocurrency and the technologies that underpin them. In some places, like the United States, regulatory bodies such as the Securities and Exchange Commission (SEC) and Financial Crimes Enforcement Network (FinCEN) have laid down stringent rules focusing on compliance and consumer protection. Meanwhile, in regions like Switzerland, regulatory landscapes are relatively more favorable towards cryptocurrency innovation, offering a more inviting environment for tech development.
- Countries with Strong Regulations:
- Countries with Flexible Regulations:
- United States
- European Union
- United Kingdom
- Switzerland
- Singapore
- Malta
These international regulatory approaches can either facilitate or hinder the adoption of AI technologies designed for fraud detection in cryptocurrency. The challenge lies in harmonizing these regulations across diverse jurisdictions to create a seamless environment that not only fosters innovation but also ensures adequate protection for investors and the market at large.
Impact of Compliance on AI Integration
Compliance has a multi-faceted impact on AI integration within the cryptocurrency sphere. When regulatory frameworks are adhered to, companies must structure their AI systems in ways that prioritize transparency, security, and accountability. For example, AI algorithms need to be explainable to regulators to ensure they meet the standards set out for ethical practices. These practices often involve the detection of suspicious patterns, identifying anomalies, and helping to minimize risks.
- Positive Aspects of Compliance:
- Improves investor confidence
- Enhances system reliability
- Creates a level playing field for all participants
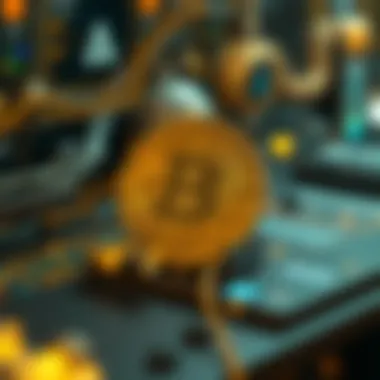
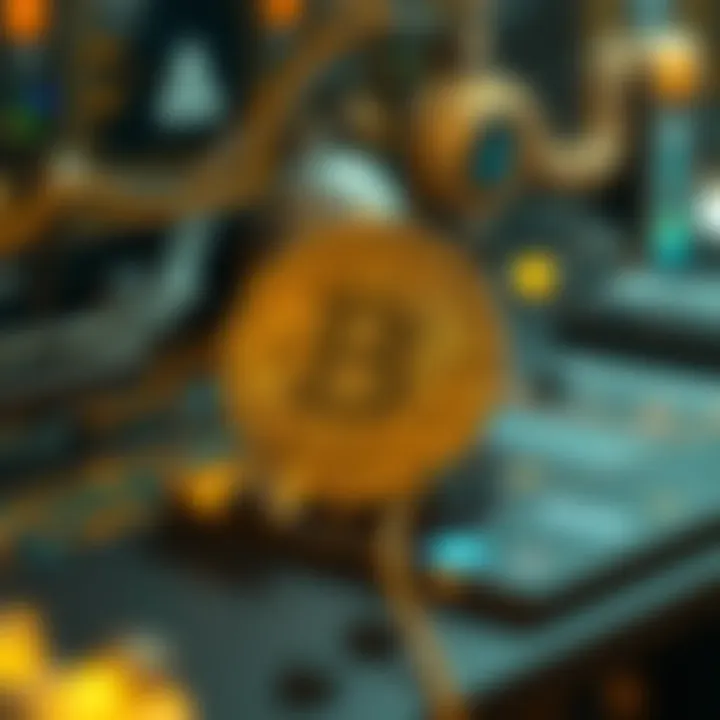
However, compliance is not without its challenges. Achieving a balance between following regulatory guidelines and fostering innovation can often feel like walking a tightrope. Excessive regulation can stifle creativity and slow down the development of new technologies needed for effective fraud detection. Companies must be agile, adapting their AI systems to meet evolving regulatory requirements while addressing market demands.
βEffective regulation can pave the way for trust and innovation, creating a safer environment for everyone involved in cryptocurrency.β
Ethical Considerations in AI Application
As artificial intelligence (AI) becomes an integral part of fraud detection within the cryptocurrency realm, the ethical implications of its application cannot be ignored. With great power comes great responsibility, and this adage rings particularly true in the financial sector where trust and integrity are paramount. Ethical considerations ensure that AI enhances security measures while also respecting individuals' rights and maintaining fairness.
Importance of Ethical Frameworks
Incorporating ethical frameworks into AI practices is paramount not only for compliance reasons but also for instilling confidence among users. As customers become more attuned to the digital landscape, they often scrutinize how their information is processed and utilized. Thus, the integration of ethics isnβt just a moral obligation; it is crucial for fostering trust within the community.
- Fairness: An ethical AI system should be equitable, mitigating biases that could lead to discriminatory outcomes.
- Accountability: Clear responsibility standards must be established to guide AI decisions and their repercussions.
- Privacy: Safeguarding user data is essential; without privacy, trust erodes quickly.
"In the world of finance, ethical considerations are not a luxury. They are necessities rooted in societal expectations."
Bias in Algorithms
Bias in algorithms is a significant concern in AI applications. These biases often originate from the data sets used to train AI models. If the data reflects existing prejudices or is unbalanced, the AI can perpetuate these discrepancies. For instance, if a fraud detection algorithm has been primarily trained on data from one demographic, it may inaccurately identify fraudulent behavior among others.
Tackling Bias
To diminish algorithmic bias, itβs essential to:
- Diverse Data Sets: Utilize varied data sources that accurately reflect a broad spectrum of behaviors.
- Regular Audits: Conduct frequent evaluations of AI outputs to identify and rectify instances of bias.
- Inclusive Development Teams: Involve individuals from different backgrounds during the AI development process to catch potential biases early on.
Transparency and Accountability
Transparency is another cornerstone of ethical AI usage, especially in fraud detection. Users need to understand how decisions are being made by AI systems. If an algorithm flags a transaction as fraudulent, the rationale behind that decision should be clear.
Establishing Clear Protocols
- Explainable AI: Developing AI systems that can articulate their decision-making process helps build transparency. Users should be able to grasp why a particular action was taken.
- Accountability Mechanisms: Organizations must outline who is responsible when AI errors occur. This includes creating frameworks that address potential grievances and corrections promptly.
Challenges Encountered in AI Deployment
The introduction of artificial intelligence into the cryptocurrency landscape has the potential to drastically enhance fraud detection mechanisms. However, realizing this potential is not without its hurdles. Itβs crucial to explore the challenges that organizations face when deploying AI systems in fraud detection. This understanding not only highlights the barriers to effective integration but also underscores the consideration that stakeholders must take into account when developing solutions.
Data Quality and Availability
One of the cardinal challenges in deploying AI for fraud detection within cryptocurrency is the quality and availability of data. For AI algorithms to function effectively, they require vast quantities of accurate and reliable data. In many cases, historical transaction data is fragmented, inconsistent, or simply unavailable, especially in a sector where anonymity can be a fundamental premise.
Consider the fact that many cryptocurrency transactions are anonymous, making it difficult to trace patterns that could indicate fraudulent behavior. Inadequate data can lead to poor model performance and an increase in false positives. For instance, if an AI system is trained on inaccurate data, it might flag legitimate transactions as fraudulent, creating inconvenience for users and mistrust in the system itself.
Furthermore, data must also be regularly updated to reflect the ever-evolving nature of fraudulent tactics in the cryptocurrency space. This is where the need for robust data management systems comes into play. Effective systems can help organizations compile comprehensive datasets, resulting in more efficient AI models that can accurately identify suspicious activities.
Integration with Existing Systems
Another significant challenge is the integration of AI technologies with existing systems. Many organizations have legacy systems in place that were not designed with advanced AI algorithms in mind. This can often lead to compatibility issues that stymie the deployment of effective AI solutions.
To improve fraud detection, AI must coexist and communicate seamlessly with various systems already in use, such as transaction monitoring systems and customer relationship management software. A failure in integration can lead to data silos, where valuable information is trapped in disparate systems, leading to efficiency losses, delayed responses to fraudulent activities, and ultimately, susceptibility to fraud.
Further complicating this integration is the need for collaboration among different teams within an organization. Technical, compliance, and operational teams must align their goals and processes to successfully implement AI-driven solutions. Training staff on new technologies is equally important, as the workforce needs to be on-board with the new systems in order to utilize AI capabilities effectively.
In summary, while AI has the potential to revolutionize fraud detection in the cryptocurrency space, the deployment comes with significant challenges, particularly around data quality and integration with existing systems.
As organizations navigate these challenges, they must adopt a holistic approach incorporating technological, operational, and human aspects to create effective fraud detection strategies.
Future Trends in AI and Fraud Detection
As the cryptocurrency landscape evolves, the integration of artificial intelligence (AI) in fraud detection becomes more critical. This section will shed light on the future trends likely to shape this intersection. Understanding these trends not only helps investors and tech professionals stay ahead but also allows marketers to craft more precise strategies, keeping the integrity of financial systems at heart.
Emerging Technologies in Fraud Prevention
New technologies are entering the fray, significantly enhancing how fraud is detected in the cryptocurrency sphere. Innovations such as blockchain analytics, biometric verification, and federated learning are gaining traction.
- Blockchain Analytics: By continuously monitoring blockchain activity, companies can use AI algorithms to detect anomalies quickly. These algorithms analyze transaction patterns and flag unusual ones, serving as an early warning system for potential scams or illicit activities. It's like having a watchful eye that never blinks.
- Biometric Verification: Moving away from traditional password systems, biometric technology leverages unique human traits, like fingerprints or facial recognition, to authenticate users. This added layer of security drastically reduces the chances of fraudulent account access. For example, using a fingerprint scanner, a user can ensure that only they can conduct transactions, making it much harder for fraudsters to infiltrate systems.
- Federated Learning: This is a novel approach where models are trained across many decentralized devices without exchanging sensitive data. Only updates to the modelβs parameters are shared with a central server. This technique not only safeguards user privacy but enhances fraud detection by pooling insights from diverse data sources, thus improving algorithm efficiency.
"Emerging technologies in fraud prevention are like having a digital fortress; they're building walls that are harder to breach."
Predictions for AI in the Cryptocurrency Sector
As we look to the horizon, several predictions can be made regarding AI's role in the cryptocurrency sector.
- Increased Automation: Over the next few years, expect a wave of automation in fraud detection processes. More institutions will adopt AI-driven solutions for monitoring transactions, which will lead to quicker responses to potentially fraudulent activities without the constant need for human input.
- Advanced Predictive Analytics: Future AI models will not just react to fraud but anticipate it. Using historical data and predictive analytics, algorithms can forecast unusual patterns. They can flag activities that typically precede fraud before it actually happens, giving organizations a chance to intervene early.
- Integration of Social Media Data: Insights from social media platforms provide a rich source of information. The future will likely see AI systems integrating social media sentiment analysis, which will help in assessing risks based on user behavior and communications that might indicate fraudulent intentions.
- Regulatory Compliance: As the regulatory landscape tightens, AI will become a key player in ensuring compliance. Smart algorithms will evolve to adapt to new regulations, helping organizations not only protect themselves against fraud but also stay on the right side of the law, thus reducing legal risks.
- Improved User Education: As AI systems become more sophisticated, there will also be a push for greater user awareness. AI will be used to educate cryptocurrency users about security practices and emerging threats, increasing overall resilience against fraud. Marketers must tailor their approaches to tap into this growing awareness and educate clients.